Daniël Vos
PostDoc at Delft University of Technology
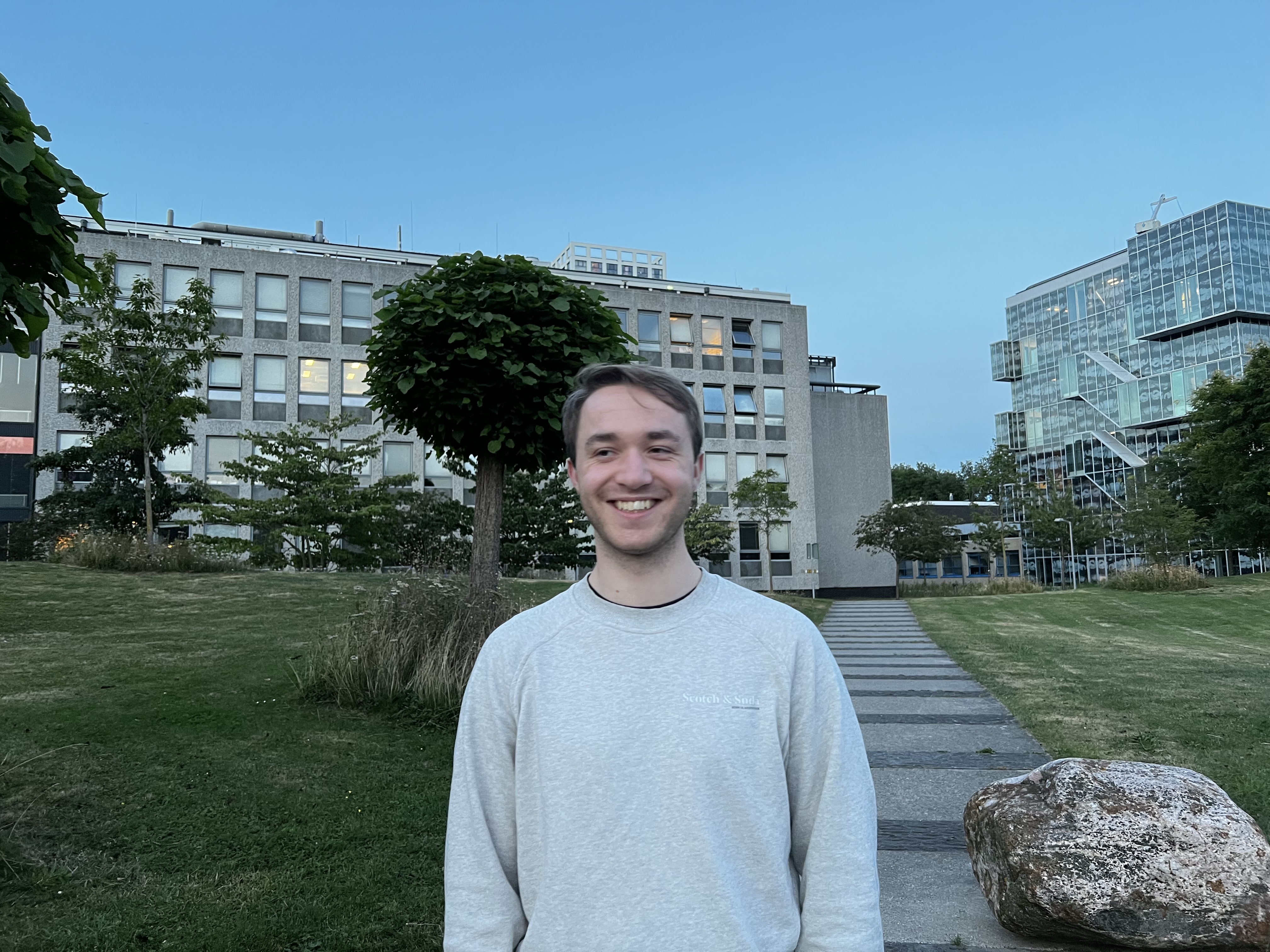
Room 4.E.420 / Building 28
Van Mourik Broekmanweg 6
2628 XE Delft
The Netherlands
Hi, I’m Daniël, a PostDoc at the algorithmics research group at the Delft University of Technology. My research is mainly on the trustworthiness of machine learning models, and I have a special fondness for decision tree models. I particularly enjoy working on the discrete optimization problems that appear when training and verifying decision tree models. For example, with our method ROCT we turned the problem of training robust decision trees against adversarial examples into combinatorial optimization which can then be solved with MILP or MaxSAT solvers. In our project OMDT, we were able to find optimal decision tree strategies for Markov Decision Processes (MDPs).
I strongly believe that we should be able to understand machine learning models that we deploy for critical applications. I worry that typical neural networks using post-hoc XAI methods give us a false sense of understanding. Therefore, I am also working on methods to train extremely small models with performance similar to that of more massive ones. My goal is to make every model as simple as possible.
Next to my academic life, I love strength training, rowing, and field hockey. I also organize Delft’s hacking team, currently one of the highest-placed teams from the Netherlands on CTFTime.